Speech Steven Maijoor - Data Science for Supervision: What’s in it for us?
Published: 13 May 2022
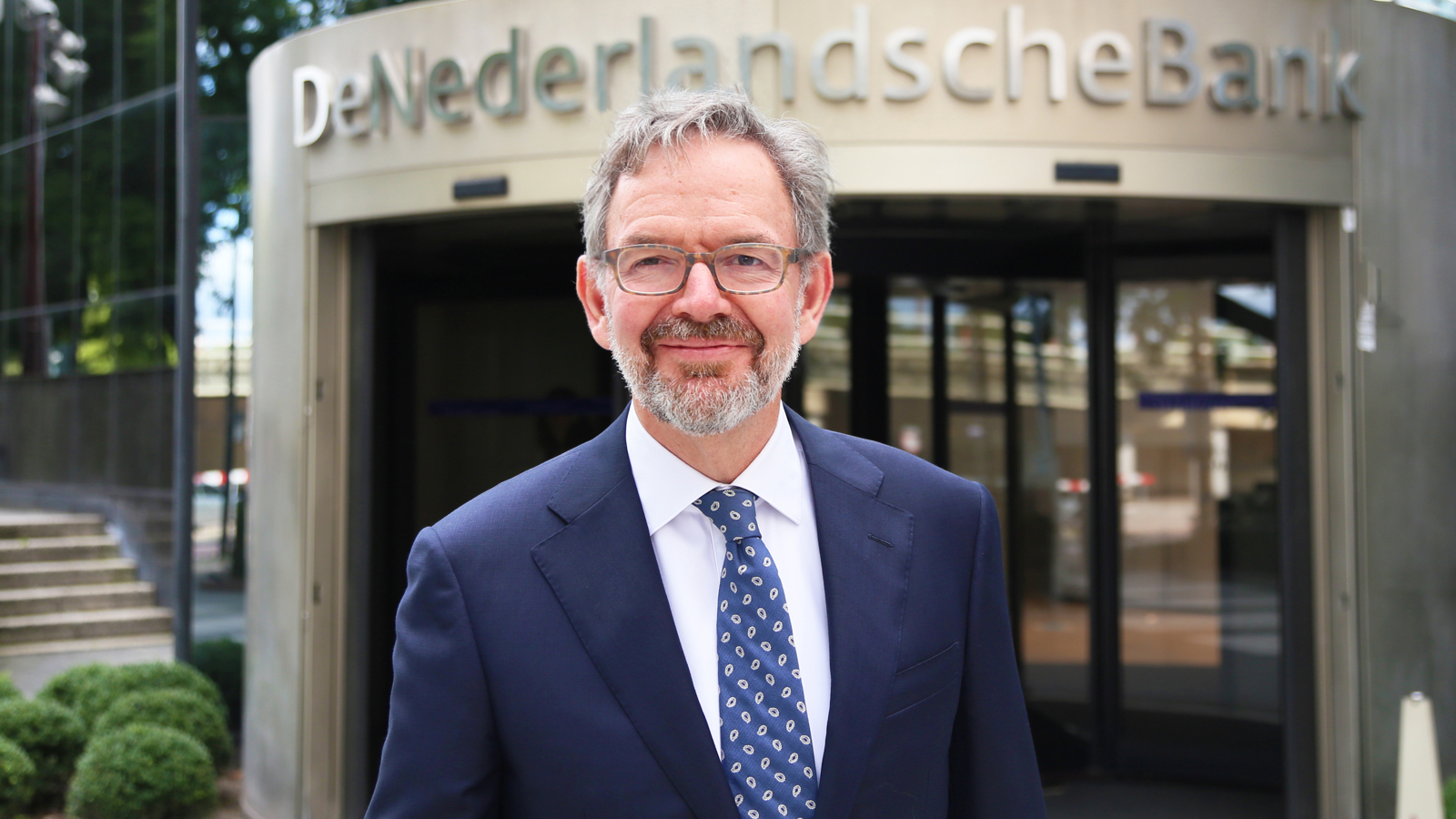
© DNB
How can supervisors leverage data even better in supervision? This is the key question in Steven Maijoor’s speech at the Data Science Conference of the DNB Data Science Hub.
Date: 13 May 2022
Speaker: Steven Maijoor
Location: Data Science Conference of the DNB Data Science Hub
I am very sorry not to be able to talk to you in person. I’m in beautiful Athens – the cradle of logical reasoning and math. Academic fields that should be close to your heart. But I have been assured that you are meeting in an equally beautiful location and are well-catered.
You are very close to Amsterdam Central station; one of the busiest railway stations in the Netherlands. As data scientists you probably immediately think of all the possibilities data science offers for the railway industry. There is even such a thing as the “Internet of Trains”. It’s a term describing the benefits that big data brings to the railway industry, making trains more efficient, more reliable and less harmful to the environment. Its main goal is to achieve a close to 100% reliability. In other words: making sure that trains are almost never late. Similarly, banking supervisors have the goal to ensure trust in the financial sector – that is a very low probability of default. And like in the railway industry, there is a huge potential of data and data science in our area of work.
As a long-time supervisor I’ve witnessed up close how supervision has been changing in the past fifteen years since the Global Financial Crisis (GFC). That crisis gave a big push to the collection of data to fill the ‘data gaps’ that existed. We now have data on nearly all parts of the financial system, including, for example, derivatives use, securities holdings and collateralised finance. In addition, a wide range of new techniques and computing power allow us to implement entirely new approaches. Taken together this allows us to do the same things better and smarter, to look into entirely new things and to do all of this this at lower cost.
Let me explain these three win-wins that new data combined with knowledge of data science can offer us.
Firstly, more granular data and data science enable us to do the same things better and smarter. Supervision has a long tradition of using quantitative data to assess risks. Having more granular data at our disposal allows us to assess, for example, concentration risks much better. We are now also better able to develop models to challenge those developed in the industry, which can make our supervision more efficient. I will return to an example of this in a minute: I will be discussing an outlier detection tool that we have applied with some success in Know Your Customer examinations at several banks. Also, Natural Language Processing (NLP) allows us to process written documents. With the help of NLP we can filter the most relevant information from the ever increasing flow of written information.
Secondly, we can also look into entirely new things. The combination of having more granular data and new data science techniques opens up a new world. Thanks to digitalisation, data is collected and available at a higher frequency. How often do you Google something? Probably quite often. The average person conducts three to four searches each and every day! This obviously generates a lot of data. Techniques such as webscraping allow us to use this data, for example, to measure market sentiment and implement this is in economic forecast models to make them real-time and more accurate.
Thirdly and lastly, we could potentially do all of this at lower cost. The cost of computing power and storage has gone down dramatically over time. Developing applications has become easier and, once implemented, could reduce personnel cost. Moreover, as the projects initiated by the BIS Innovation Hub show, its participants are keen to develop functionality together. This could lead to significant efficiency goals because – instead of sharing shiny PowerPoint presentations – we could share the functionality that allows us to replicate each other's analyses with our own data.
Now, this conference aims to explore how new techniques can help financial authorities – that is, both supervisors and central bankers – to improve and to learn. And this is close to my heart: also during my previous role at the European Securities and Markets Authority, (ESMA), I’ve always been a keen supporter of increased data-driven supervision. And data-driven supervision starts with good data. And let me stress that data should be fit-for-purpose: ideally, we all want flawless information. I have seen first-hand the amount of effort and tenacity required to achieve that. However, timeliness also has value. Especially in this dynamic world in which unforeseen events like the COVID-19 pandemic and the war in Ukraine have immediate impact on the economy and the financial sector. For timely policy making, immediacy is then more important even if it might come at the cost of accuracy. There are, however, quick wins when it comes to data quality.
Let me start by saying that labelling data is key, and I will give you an example. The GFC has shown us that exogenous risks – triggered by external events – are not the only risks in the financial system. Endogenous risk, generated and reinforced by financial institutions acting as part of the financial system, has shown to be as important, if not more important. That was illustrated for example with securitisations and derivatives. The increased interconnectedness and complexity within the financial system increases the importance of improved insight in the existing interlinkages and potential spill-over effects. To get a complete overview of the interlinkages, and thereby of systemic risks, one must be able to uniquely identify institutions. That is exactly why the Legal Entity Identifier – or LEI – was introduced as a global standard. However, adaption and implementation is still relatively limited, which is a pity: The more entities obtain an LEI, the greater the benefits are in terms of getting this complete overview of the financial system.
Let me turn now from data to the part that is more relevant to this conference: data science. What do I expect the techniques that you are discussing to deliver? To phrase it in the simplest words: my hope is that you can replicate my WhatsApp experience. Just like many of you, I use this app a lot to communicate, and I’m impressed by – and a little bit scared of – its predictive text options. It has the uncanny ability to guess what it is I want to type next. I do not pretend to understand the underlying technical intricacies but the intuition is clear to me: by continuously correcting it, I allow the system to learn what my preferred combination of words is. The algorithm can then help me communicate more easily and more efficiently.
What I would hope is that the methods discussed in these two days can help supervisors in a way similar to what WhatsApp has done for my communication. So, amongst other things:
- Algorithms should capture relevant aspects of the supervisory process and help us deliver a safer financial system.
- Algorithms should learn to capture anomalous payments that can be linked to illicit activities.
- Algorithms should help us gauge market reactions to monetary policy interventions.
In all cases, the algorithms should support policy makers and supervisors to arrive at better decisions.
I'm aware that this technology is not entirely free of new problems. Or, put more optimistically, challenges. For instance, the algorithm could exhibit biases and lead to discriminatory outcomes. Also, as with any data-dependent method, it is inherently backward-looking since it takes time to collect data and train the models. Furthermore, since many of the techniques are new, users often feel they are forced to use black boxes with very low explainability. Up to a point, they are right, since approaches are often a-theoretical, making it difficult to establish not just correlation but also causality. Therefore, more and more work is being done to create ‘Explainable AI’. Lastly, since we often have to turn to cloud-based implementation – primarily because of computational demands – data security is an issue. I don’t want to sweep these issues under the rug, but I do feel that careful application can yield benefits and that the least we can do is explore the possibilities.
These possibilities have implications for how we supervise. In terms of supervision – the topic closest to my experience – these developments will have a significant impact on how we work.
Let me first turn to a showcase of how we, at De Nederlandsche Bank, have applied these new techniques in actual supervision.
In the last year we have employed an outlier detection algorithm in “know your customer”-deep dives at several institutions. As you all may know, a great deal of media attention is given these days to preventing financial institutions from being used by criminals for activities such as money laundering. The standards that were designed to protect these institutions against money laundering were implemented in 2012 and are also referred to as “Know Your Customer” or KYC in short. Financial institutions have to comply with the standards, and as a supervisor, De Nederlandsche Bank needs to ensure that banks have incorporated the minimum standards in their business operations and that their systems work properly in fighting financial crime.
But how to detect potential fraudulent transactions in a dataset that contains millions of customers and bank accounts and billions of transactions? Data science has a clear role to play here. To identify exceptions we applied an Isolation Forest algorithm, and I probably don’t need to explain it to this audience. But please indulge me while I take a few minutes to explain to you what I take away from the intuition and workings of an algorithm like this.
Since we have limited resources and since our supervision is risk based, we are looking for the most unlikely combinations across millions of account holders with billions of transactions. To find these exceptions, we would traditionally define tell-tale identifiers. For example, “multiple accounts on a single address” and “a single deposit per month and immediate withdrawal”. Seen separately these are relatively innocent. Together, however, they can indicate human trafficking of seasonal workers: the combination identifies subcontractors who organise housing for seasonal workers – which is a perfectly legal activity – but then at the same time immediately withdraw the wages deposited and only pay a fraction to the worker – which is illegal off course. However, this combination could to an extent also identify student housing: a large inflow when student grants and loans arrive and a relatively quick withdrawal rate. These are just two dimensions. In practice, there are many and these interact in non-linear and unpredictable ways. With the use of data science techniques we can identify those. Our showcase has proved itself already: my colleagues in integrity supervision can now do their work in a more efficient manner by selecting the most risky files using data science.
So what can we learn from this example? Let me draw a few conclusions:
Data Science has great potential, and data scientists can definitely add value. The data scientists should however work closely with the business – that is the supervisors with the business knowledge. This is not only needed for providing input to the model, but also for interpreting model outcomes. Considering the example I just gave; while the algorithm flags fraudulent transactions that would otherwise never have been identified, left to its own devices the model is not – or not yet – able to make a distinction between illegal activities and student housing.
How can you make sure that data science knowledge is always combined with business knowledge? In organisational terms this is a challenge: if you embed data science locally, how can you keep the data science knowledge up to date? Conversely, if you set up data science centrally, how do you prevent empire building? At De Nederlandsche Bank, we chose a hub-and-spoke system for this – stressing joint development. Our data scientists work in a Data Science Hub, together with the spokes – which are the various divisions of De Nederlandsche Bank. This comes with the additional benefit of making optimal use of potential spill-overs – using the same code for different applications - while at the same time working on a variety of topics, from financial markets and pension funds to payment services and even Human Resources.
So far so good, but if one wants to embed the data science tools, such as the outlier detection tool, into existing workflows there is the challenge of correctly implementing and, subsequently, maintaining it. How to implement and maintain the tool? The traditional approach is to deliver a data science solution to an IT department as a Proof of Concept to implement in production. Often, this has the drawback of a longer time to market and a loss of flexibility. On top of that, there is a need to maintain the tool. Not only in a technical sense – ensuring that it remains operational – but also in a more practical sense. What if the model requires updates due to changes in the underlying data or processes? In those cases in which models are embedded into workflows, there may be a need for a stronger collaboration between the business, the developers and operations. An alternative approach with which we are experimenting is something called BizDevOps. A type of organisation I probably don’t need to explain, but that encourages collaboration between these three parties: the business, the developers and the operations team, by combining development and operations in a single team. We are not yet sure whether this provides an optimal solution, but we believe it’s worth a try.
With this, I have shared some of the experiences we have gained at De Nederlandsche Bank. I am glad to see that offline conferences are back, and I invite you to make the best possible use of this and share tips and tricks during these two days. I’m especially interested to know what the speeddate session you had before lunch will bring you. We are probably all in the same phase of experimenting with data science within central banking and supervision. Let’s share not only code via Github but also experiences in this offline environment. Let me also mention the Innovation Forum – or iForum – through which we want to strengthen the interaction with the financial ecosystem in the context of technological innovations. The iForum aims to move beyond just comparing notes; pilots and experiments are a core activity and maybe some of the ideas presented here can find their way to the iForum.
So, let me come to a close. For me, as a supervisor it is key that the marvellous insights presented here are in the end implemented. Only when we change work processes for the better we will have a real and meaningful impact. Looking at the topics and discussions at this conference, I have no doubt that we will be successful.
Discover related articles
DNB uses cookies
We use cookies to optimise the user-friendliness of our website.
Read more about the cookies we use and the data they collect in our cookie notice.